In today’s manufacturing landscape, data is not just a byproduct of operations—it’s a transformative asset that can drive decision-making, innovation, and business transformation, inspiring a new era of manufacturing excellence.
Every machine, sensor, process, and transaction generates data, creating a rich tapestry of information that, if utilized correctly, can unlock unprecedented insights and efficiencies.
However, the reality in many manufacturing organizations is that data in manufacturing often remains siloed and underutilized.
Enterprise Resource Planning (ERP) systems, Industrial Internet of Things (IIoT) devices, ad-hoc Excel sheets, and other sources generate data assets that are rarely integrated into a cohesive framework for decision-making.
Instead of fully leveraging the power of data analytics, organizations often find themselves overwhelmed by data overload, struggling to distill meaningful insights from a multitude of disparate data sources.
For CEOs, this poses both a challenge and an opportunity. This white paper aims to explore how manufacturing organizations can shift from mere data collection to strategic data utilization, using data dashboards and predictive data to drive more informed, real-time, and forward-looking decision-making. The goal is to offer a deep dive into the key aspects of data effectiveness, data analytics, and the practical application of predictive data in manufacturing.
Content
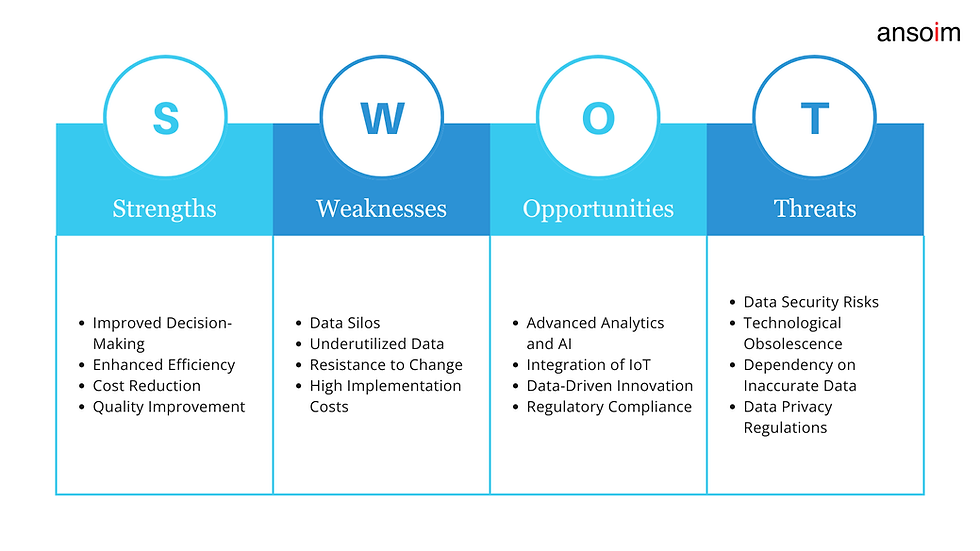
The Growing Complexity of Data in Manufacturing
Manufacturing environments are inherently data-rich. Every aspect of the operation—from supply chain management to shop floor production, to quality control and maintenance—generates an array of data points that, when aggregated, tell a story about operational performance, bottlenecks, and potential areas for improvement. However, the complexity of this data is one of the biggest barriers to extracting value.
Consider the primary sources of data in manufacturing:
ERP systems: ERP provide structured, transactional data related to procurement, inventory, production schedules, finances, and human resources. They are the backbone of many organizations but often offer little in terms of real-time or predictive insights.
IoT devices: The rise of IIoT allows manufacturers to collect granular, real-time data from sensors embedded in machines and processes. This data is often continuous but fragmented, creating a challenge when integrating it into broader operational datasets.
Excel and ad-hoc reports: Despite technological advancements, many organizations still rely on manual spreadsheets and ad-hoc reporting for critical business decisions. While flexible, this form of data is often unstructured and lacks the scalability needed for comprehensive analysis.
The challenge is clear: integrating these various data sources into a single, cohesive system that can provide real-time, actionable insights.
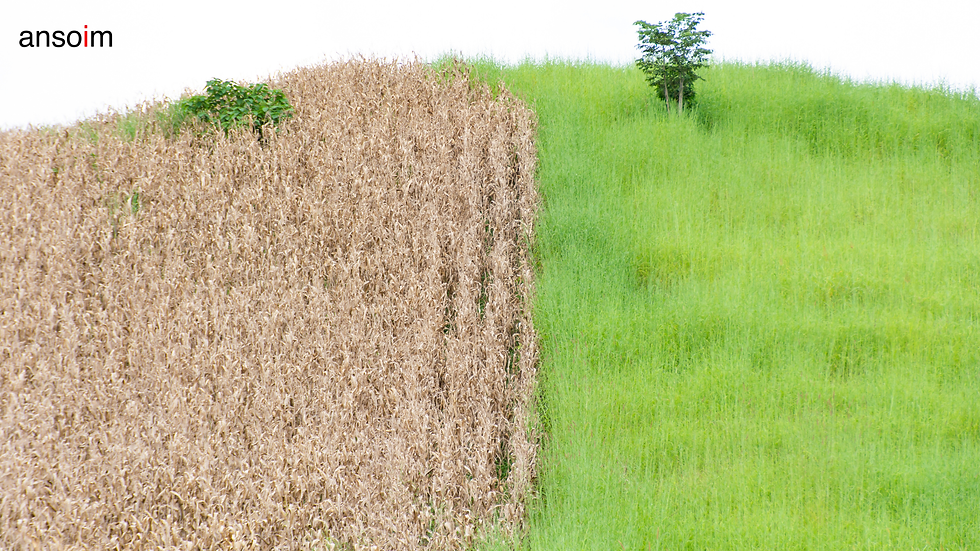
Data Effectiveness: Turning Data Overload into Strategic Insight
The key to overcoming data overload lies in a concept known as data effectiveness—the ability to transform raw data into valuable insights that can directly inform business decisions. But this transformation doesn’t happen by accident. It requires a structured approach, grounded in clear business objectives and supported by robust technological infrastructure.
Step 1: Define the Strategic Objectives
Prior to delving into data integration or analytics, organizations must first establish their strategic objectives. Whether the aim is to minimize machine downtime, optimize supply chain efficiency, or enhance overall productivity, clearly defined objectives should shape the organization’s data strategy.
For example, a manufacturing CEO may prioritize the following:
Improving machine uptime: By integrating IoT data with ERP systems, manufacturers can monitor real-time machine performance and identify bottlenecks and inefficiencies that contribute to downtime.
Optimizing quality control: Data analytics can uncover patterns in production data that predict defects before they occur, enabling proactive adjustments that improve product quality.
Supply chain optimization: Predictive analytics can be applied to procurement and inventory data, forecasting potential disruptions in the supply chain and allowing manufacturers to adjust production schedules accordingly.
The critical point here is that data effectiveness is not about collecting more data—it’s about collecting and analyzing the right data in pursuit of specific business outcomes.
Step 2: Integrate Data Across Multiple Platforms
Once strategic objectives are defined, the next step is data integration—consolidating data from multiple disparate sources into a unified framework. This is often the most challenging aspect of data analytics in manufacturing, but it's a crucial step that ensures the effectiveness of your decision-making processes.
Here’s how leading manufacturers achieve seamless data integration:
ETL (Extract, Transform, Load): ETL tools extract data from sources such as ERP systems, IoT devices, and Excel spreadsheets, transform it into a standardized format, and load it into a centralized database. This allows for a single source of truth where all data is consolidated and ready for analysis.
Data Lakes: These large repositories can store structured and unstructured data from a variety of sources. By housing all data in one location, companies can perform both real-time and historical analyses, enabling them to look at trends over time or react to current operational conditions.
API Integration: APIs (Application Programming Interfaces) enable real-time data sharing between different platforms, making it easier for IoT sensors, ERP systems, and even external suppliers to communicate and share data in real time.
Effective data integration allows CEOs and data analysts to move away from siloed decision-making and toward a more comprehensive view of the entire manufacturing ecosystem.
Step 3: Data Standardization and Cleaning
Integrating data is only the first step. To ensure that data analytics yield accurate and actionable insights, the data must be cleaned and standardized. This involves removing inconsistencies, correcting errors, and ensuring that all data is presented in a uniform format.
For example:
Inconsistent Units of Measurement: One plant may report machine uptime in hours, while another reports in MTBF. Standardizing these metrics is crucial to making apples-to-apples comparisons across the organization.
Incomplete Data: Gaps in data, such as missing sensor readings or incomplete inventory records, must be addressed to avoid skewing analytics results.
Data cleaning is an ongoing process, especially as organizations continue to collect new data from various sources. However, without this crucial step, even the most sophisticated analytics tools will struggle to produce reliable insights.
Step 4: Applying Advanced Data Analytics
Once data has been integrated and cleaned, it’s time to apply advanced data analytics to uncover insights that were previously hidden beneath the surface. This is where the true value of data in manufacturing begins to emerge.
Advanced analytics go beyond simple reporting or descriptive statistics. They allow organizations to explore why certain trends are happening and how they can take action to influence future outcomes.
Here are some of the key techniques:
Descriptive Analytics: This form of analysis focuses on understanding historical performance by summarizing key metrics. For example, examining production line efficiency over the last quarter or week to identify which lines are performing above or below expectations.
Diagnostic Analytics: This dives deeper into root causes. Why is one production line consistently underperforming? Diagnostic analytics can identify patterns and correlations that help uncover the reasons behind certain outcomes.
Predictive Analytics: Perhaps the most transformative aspect of data analytics in manufacturing, predictive data allows organizations to anticipate future trends based on historical data. For example, predictive maintenance uses machine learning models to predict when a machine is likely to fail, allowing maintenance teams to intervene before costly downtime occurs.
Prescriptive Analytics: The most advanced form of analytics, prescriptive analytics, provides actionable recommendations based on predictive insights. This might involve adjusting production schedules or reallocating resources in real-time to prevent a predicted bottleneck or production shortfall.
The real potential for optimization and growth lies in the application of predictive data. By forecasting future trends and identifying opportunities for proactive interventions, manufacturers can shift from a reactive to a proactive operational model.
Step 5: Building a Data Dashboard for Real-Time Decision-Making
Data dashboards are the final piece of the puzzle, transforming complex data analytics into simple, actionable insights that decision-makers can easily understand. Across the hierarchy, the ability to visualize key performance indicators (KPIs) in real-time is invaluable.
When designing a data dashboard, key considerations include:
Simplicity: Focus on displaying only the most important metrics. Too much data can be overwhelming and reduce decision-making clarity.
Real-time Updates: Dashboards should update in real-time, providing leaders with an accurate, up-to-date picture of operations.
Customization: Each user should be able to tailor the dashboard to their specific needs, ensuring that they have access to the most relevant data for their role.
Drill-Down Capabilities: Beyond surface-level insights, dashboards should allow users to drill down into specific data points for more detailed analysis.
A well-designed dashboard turns complex analytics into digestible, actionable insights that enable faster, smarter decision-making at every level of the organization.
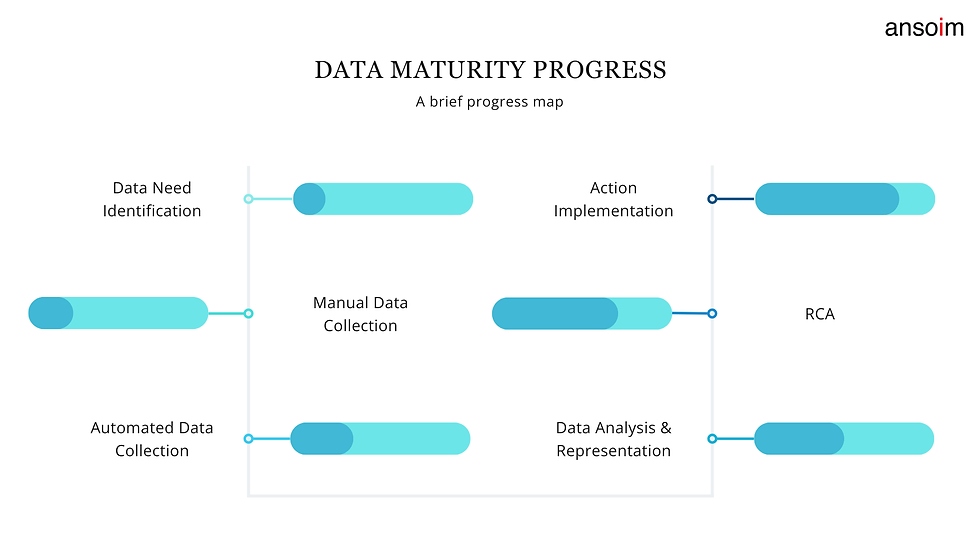
Leveraging Predictive Data for Future Success
For manufacturing leaders, predictive data is the key to unlocking future success. Rather than reacting to problems after they occur, predictive analytics allow organizations to anticipate issues before they arise, positioning them to take proactive action.
Some of the most impactful uses of predictive data in manufacturing include:
Predictive Maintenance: By analyzing sensor data from machines, manufacturers can predict when a machine is likely to fail, scheduling maintenance in advance to avoid unplanned downtime.
Demand Forecasting: Predictive models can help manufacturers forecast customer demand, allowing them to adjust production schedules and inventory levels to meet future needs.
Supply Chain Optimization: Predictive analytics can identify potential bottlenecks in the supply chain, enabling manufacturers to address disruptions before they impact production.
Manufacturers can reduce downtime, optimize resource allocation, and improve profitability by shifting from reactive to predictive decision-making.
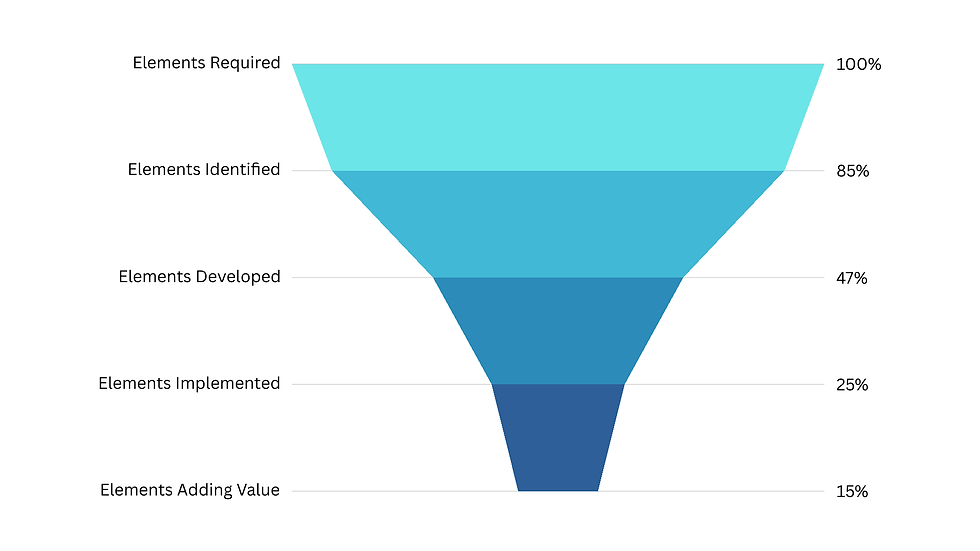
Organizational Readiness: Preparing for a Data-Driven Future
While data integration and analytics are critical, organizational readiness is equally important for a successful transformation. Without proper planning, the best technology and processes will fail to deliver their full potential.
Assessing Organizational Readiness
Before embarking on a data transformation journey, organizations must assess their readiness to adopt new technologies and processes.
Key factors to consider include:
Technological Infrastructure: Does the organization have the hardware, software, and network capabilities to support large-scale data analytics? For instance, advanced data dashboards and predictive models require robust computing power and scalable cloud infrastructure.
Data Literacy: Do employees, especially decision-makers, have the skills to interpret and act on data insights? If not, training programs will be necessary to build this capability across the organization.
Process Maturity: Are existing processes ready for integration with new data-driven workflows? Or will the organization need to overhaul outdated procedures to ensure seamless adoption?
This assessment should inform the broader data strategy, helping leaders identify gaps that need to be addressed before moving forward.
Fostering a Culture of Data-Driven Decision Making
Even the most data-rich organizations struggle to extract full value from their analytics if they don’t foster a culture that embraces data-driven decision-making.
CEOs play a critical role in setting the tone from the top, ensuring that every department—from operations to sales to HR—recognizes the importance of data in driving decisions.
This requires a commitment to data literacy and incentivizing employees to make data-backed decisions. For instance, integrating data analytics into performance metrics can encourage teams to embrace predictive insights rather than rely solely on intuition.
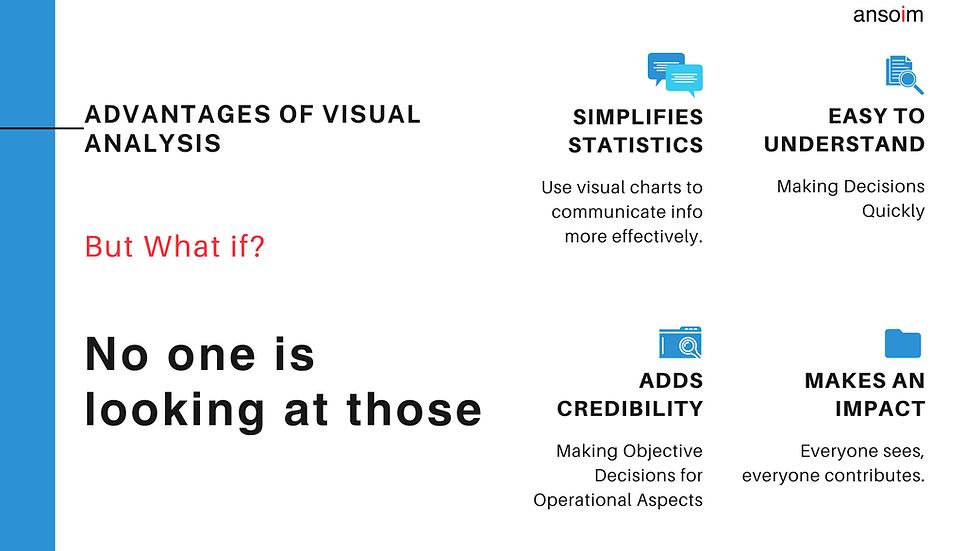
Change Management: Leading the Data-Driven Transformation
The journey to becoming a data-driven manufacturing organization isn’t simply about technology; it’s also about managing change across the organization. Change management is essential for the data transformation process.
Key Elements of Change Management for Data Analytics
Leadership Buy-In: CEOs and senior leaders must be fully committed to the transformation, championing data initiatives and setting clear expectations for success.
Clear Communication: Throughout the process, leaders must clearly and consistently communicate the transformation's benefits to all levels of the organization. Employees must understand why change is necessary and how it will impact their roles.
Training and Support: Offering comprehensive training programs helps bridge skill gaps and ensures employees feel confident using new data tools and dashboards. Support systems should be in place to address any challenges that arise as new processes are adopted.
Incremental Rollout: Rather than implementing sweeping changes all at once, organizations should adopt a phased approach, rolling out new tools and processes in manageable stages. This allows for smoother adoption and provides time to address any unforeseen challenges.
By prioritizing change management, CEOs can ensure that their organizations are not only equipped with the right data tools but are also aligned culturally and operationally to fully leverage the power of data.
Conclusion: A Data-Driven Future for Manufacturing
The future of manufacturing is data-driven. For CEOs, the challenge lies in fostering a culture of data-driven decision-making and investing in the right technology and talent to unlock the full potential of their data.
The focus should be on building the systems and processes that turn raw data into actionable insights, enabling more informed, proactive decisions at every level of the organization.
The road to a data-driven future is not without its challenges, but the rewards—greater operational efficiency, lower costs, and higher profitability—are well worth the effort.
In a world where data is power, those who harness it most effectively will lead the next wave of industrial transformation.